Details:
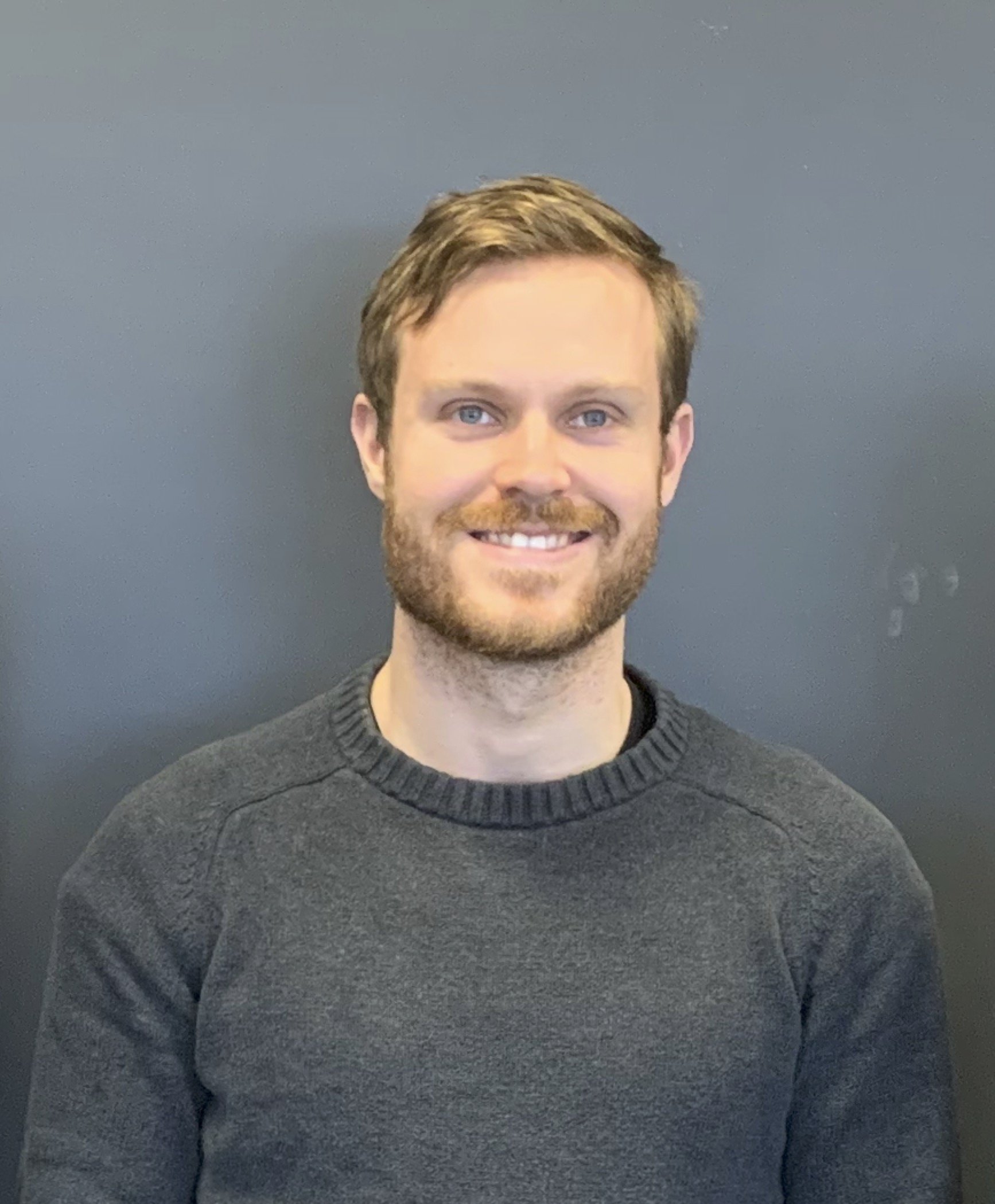
Speaker: Dr. Ted Westling (Assistant Professor, University of Massachusetts Amherst)
Title: Consistency of the bootstrap for asymptotically linear estimators based on machine learning
Abstract: The bootstrap is a popular method of constructing confidence intervals due to its ease of use and broad applicability. Theoretical properties of bootstrap procedures have been established in a variety of settings. However, there is limited theoretical research on the use of the bootstrap in the context of estimation of a differentiable functional in a nonparametric or semiparametric model when nuisance functions are estimated using machine learning. In this article, we provide general conditions for consistency of the bootstrap in such scenarios. Our results cover a range of estimator constructions, nuisance estimation methods, bootstrap sampling distributions, and bootstrap confidence interval types. We provide refined results for the empirical bootstrap and smoothed bootstraps, and for one-step estimators, plug-in estimators, empirical mean plug-in estimators, and estimating equations-based estimators. We illustrate the use of our general results by demonstrating the asymptotic validity of bootstrap confidence intervals for the average density value and G-computed conditional mean parameters, and compare their performance in finite samples using numerical studies. Throughout, we emphasize whether and how the bootstrap can produce asymptotically valid confidence intervals when standard methods fail to do so.
This is joint work with UMass Amherst Statistics PhD student Zhou Tang. A preprint of the paper is available here: https://arxiv.org/abs/2404.03064.
|